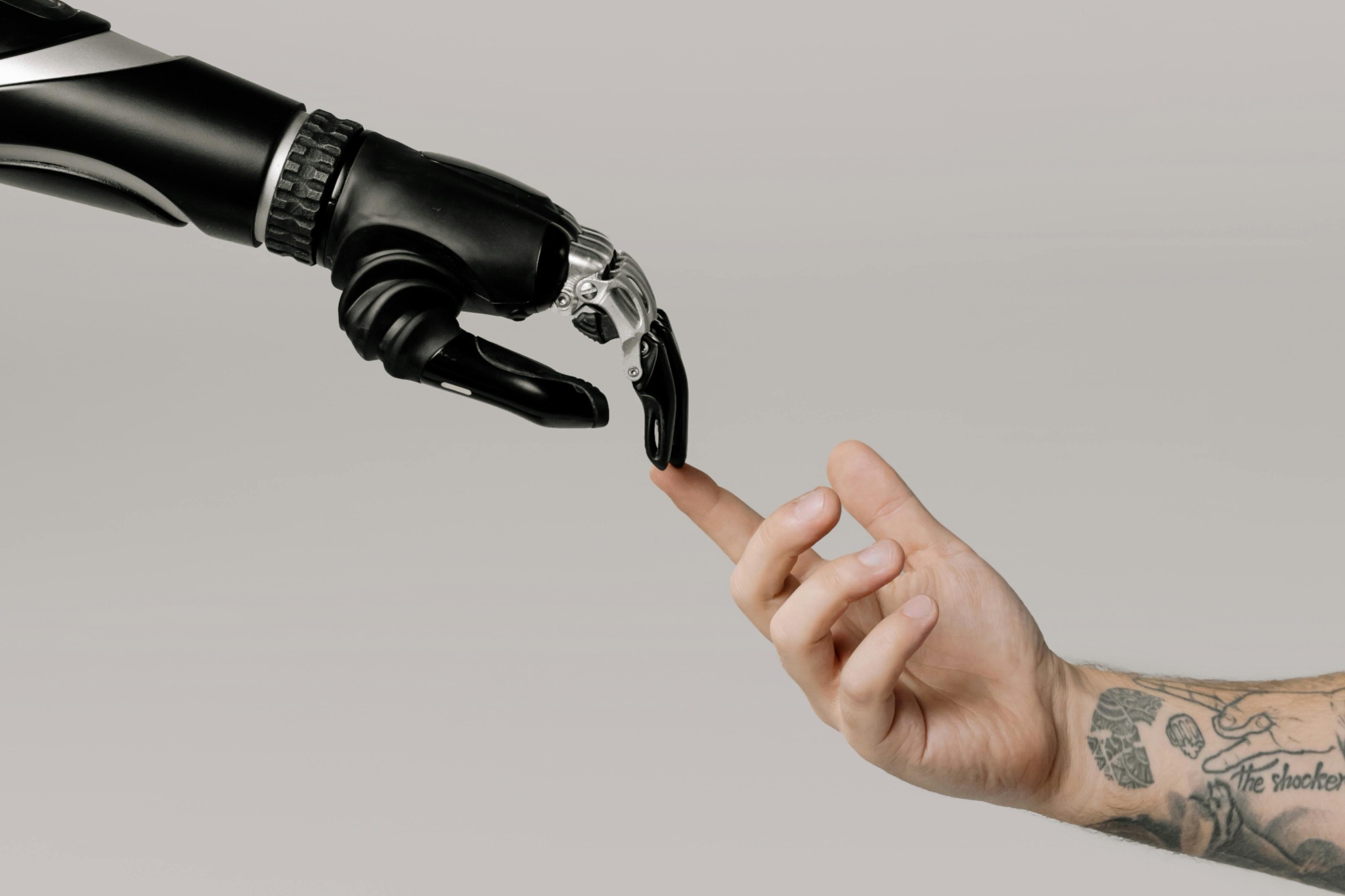
Scalable Content Solutions Using AI
The Problem
In the fast-paced world of product documentation, keeping content up-to-date, relevant, and user-friendly is a constant challenge. Our team at AWS identified a critical need to streamline the process of identifying and updating low-value or outdated content across our extensive documentation library.
How We Identified the Problem
Through a combination of user feedback, analytics data, and internal content audits, we discovered that:
- Customers were struggling to find relevant information quickly
- Outdated content was leading to confusion and support tickets
- Content creators were spending excessive time manually reviewing and updating documents
The Goal
Our primary objective was to develop an automated system that could:
- Identify low-value or outdated content
- Provide actionable insights to content creators
- Streamline the content update process
- Improve overall user experience and satisfaction
Success would be measured by:
- Reduction in time spent on manual content reviews
- Increase in user engagement with documentation
- Decrease in support tickets related to outdated information
- Positive feedback from content creators on the new system
My Role and Process
As the Content Strategist leading this initiative, I:
- Conducted in-depth analysis of existing content management processes
- Collaborated with data scientists to develop an ML model for content evaluation
- Worked with UX designers to ensure the solution aligned with user needs
- Led cross-functional team meetings to align objectives and track progress
- Developed and refined AI prompts for content analysis
- Coordinated with technical writers for testing and feedback
- Iterated on the solution based on ongoing feedback and performance metrics
Collaboration and Leadership
I fostered a collaborative environment by:
- Organizing regular sprint meetings with team members from various disciplines
- Facilitating knowledge sharing sessions between technical and non-technical team members
- Advocating for the needs of both content creators and end-users throughout the development process
Iteration Process
Our approach involved:
- Developing an initial prototype based on available data and user research
- Conducting small-scale tests with a subset of content creators
- Gathering feedback and performance metrics
- Refining the model and user interface based on insights
- Gradually expanding the scope of testing
- Continuously optimizing based on real-world usage data
Data-Driven Decision Making
We leveraged various data sources to inform our decisions:
- User engagement metrics (page views, time on page, bounce rates)
- Content metadata (creation date, update frequency, word count)
- User feedback and ratings
- Support ticket trends related to documentation issues
End-to-End Experience Consideration
Our solution went beyond just identifying problematic content. We considered:
- The content creator's workflow and how to seamlessly integrate our tool
- The impact on the overall user journey through our documentation
- How improvements in one area might affect other parts of the documentation ecosystem
- The long-term maintainability and scalability of the solution
Constraints and Trade-offs
We faced several challenges:
- Limited availability of certain types of content metadata
- The need to balance automation with human oversight
- Varying needs across different documentation types and user segments
To address these, we:
- Developed creative solutions to extract useful insights from available data
- Implemented a hybrid approach that combined AI recommendations with human decision-making
- Created a flexible system that could be customized for different content types and user needs
Outcomes and Learnings
The project was a significant success:
- We reduced the time spent on manual content reviews by approximately 40%
- User engagement with documentation increased by 25%
- Support tickets related to outdated information decreased by 30%
- Content creators reported high satisfaction with the new system, praising its ease of use and actionable insights
Key learnings:
- The importance of cross-functional collaboration in solving complex content challenges
- The power of combining human expertise with AI-driven insights
- The value of continuous iteration and user feedback in developing effective tools
- The need for flexible solutions that can adapt to diverse content ecosystems
Post a comment